Speed Comparison
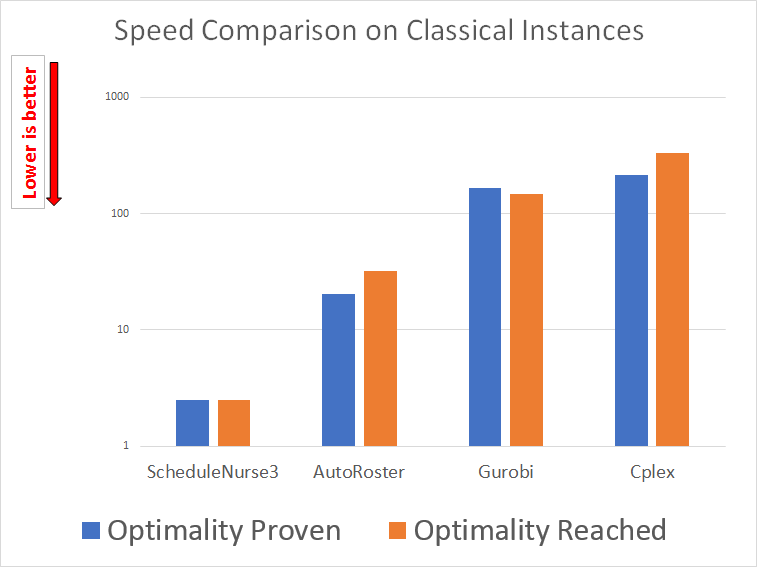
Optimality Proven Instances
Instance Name | Cplex | Gurobi | AutoRoster | ScheduleNurse3 |
---|---|---|---|---|
QMC-1 | 2.95/2.25=1.3 | 2.25/2.25=1 | - | 8.5/2.25=3.8 |
SINTEF | 1.89/0.78=2.4 | 0.78/0.78=1 | 9/0.78=11.5 | 1.15/0.78=1.5 |
ikegami-3Shift-DATA1.2 | - | 695/5.66=122.8 | - | 5.66/5.66=1 |
ikegami-3Shift-DATA1.1 | 6606/7.155=923.3 | 416/7.155=58.1 | - | 7.155/7.155=1 |
ikegami-3Shift-DATA1 | 1838/4=459.5 | 285/4=71.3 | - | 4/4=1 |
ikegami-2Shift-DATA1 | 9.23/0.14=65.9 | 0.14/0.14=1 | 11/0.14=78.6 | 1.94/0.14=13.9 |
GPOST-B | 227/34=6.7 | 161/34=4.7 | 40/34=1.2 | 34/34=1 |
GPOST | 124/2.8=44.3 | 22/2.8=7.9 | 17/2.8=6.1 | 2.8/2.8=1 |
Valouxis-1 | - | - | - | 37/37=1 |
WHPP | - | 4853/4=1213.3 | 17/4=4.3 | 4/4=1 |
BCDT-Sep | - | - | - | 140/140=1 |
Optimal Objective Reached Instances
Instance Name | Cplex | Gurobi | AutoRoster | ScheduleNurse3 |
---|---|---|---|---|
QMC-1 | 2.95/2.25=1.3 | 2.25/2.25=1 | 140/2.25=62.2 | 8.5/2.25=3.8 |
SINTEF | 1.89/0.78=2.4 | 0.78/0.78=1 | 9/0.78=11.5 | 1.146/0.78=1.5 |
ikegami-3Shift-DATA1.2 | 2573/4=643.3 | 184/4=46 | - | 4/4=1 |
ikegami-3Shift-DATA1.1 | 6606/3.94=1676.6 | 175/3.94=44.4 | - | 3.94/3.94=1 |
ikegami-3Shift-DATA1 | 1200/4=300 | 285/4=71.3 | 300/4=75 | 4/4=1 |
ikegami-2Shift-DATA1 | 9.23/0.14=65.9 | 0.14/0.14=1 | 11/0.14=78.6 | 1.94/0.14=13.9 |
GPOST-B | 130/2.5=52 | 61/2.5=24.4 | 40/2.5=16 | 2.5/2.5=1 |
GPOST | 124/2.3=53.9 | 22/2.3=9.6 | 17/2.3=7.4 | 2.3/2.3=1 |
Valouxis-1 | 663/3.91=170 | 224/3.91=57.3 | 9/3.91=2.3 | 3.91/3.91=1 |
WHPP | - | 4853/4=1213.3 | 17/4=4.3 | 4/4=1 |
BCDT-Sep | - | - | - | 140/140=1 |
Time - Number of Instances proven optimality
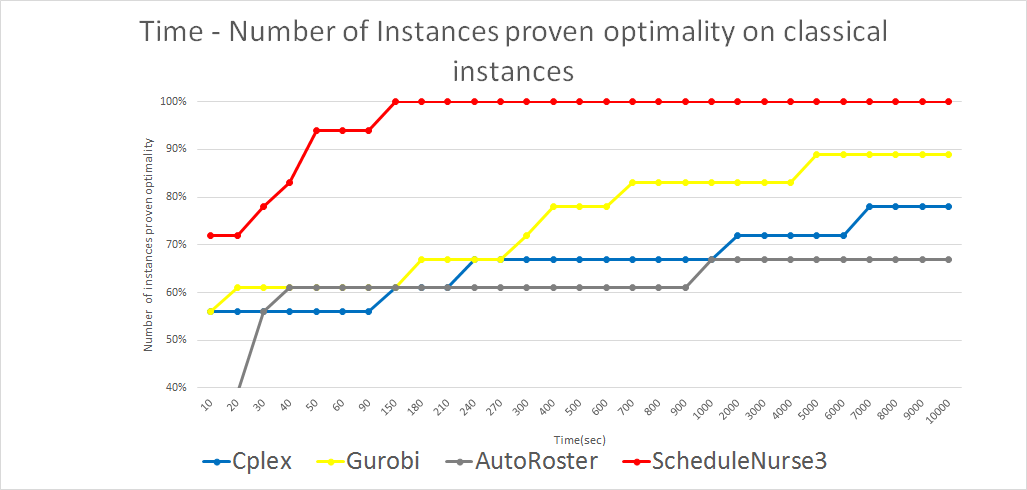
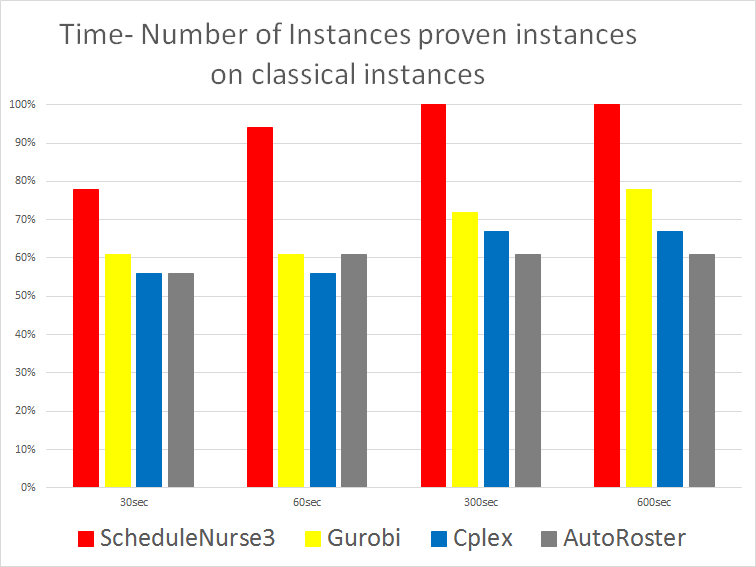
No. | Instance Name |
---|---|
1 | Millar-2Shift-DATA1.1 |
2 | Millar-2Shift-DATA1 |
3 | Ozkarahan |
4 | Musa |
5 | Azaiez |
6 | QMC-1 |
7 | LLR |
8 | SINTEF |
9 | ikegami-3Shift-DATA1.2 |
10 | ikegami-3Shift-DATA1.1 |
11 | ikegami-3Shift-DATA1 |
12 | ikegami-2Shift-DATA1 |
13 | GPOST-B |
14 | BCV-4.13.1 |
15 | GPOST |
16 | Valouxis-1 |
17 | WHPP |
18 | BCDT-Sep |
Time - Number of Instances reached optimal objective
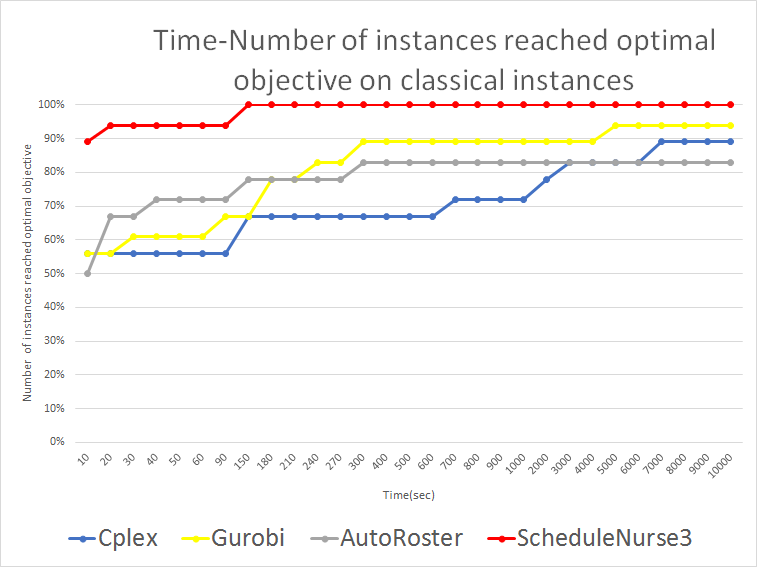
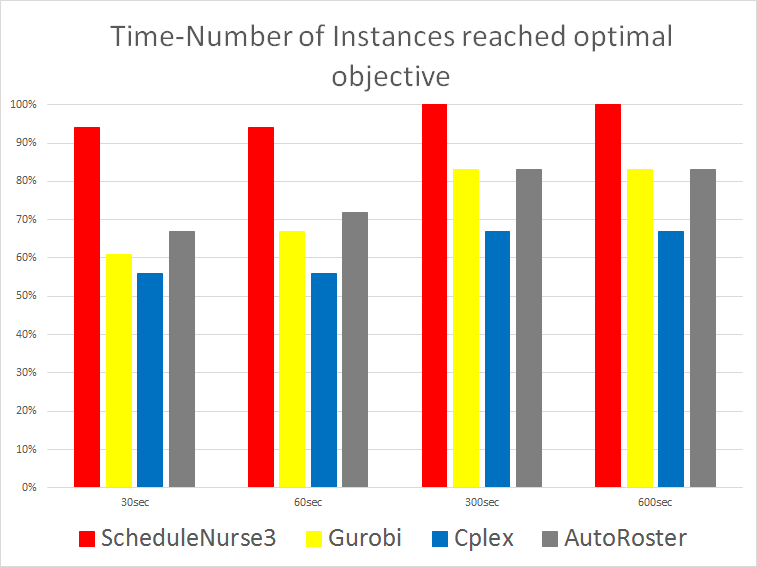
No. | Instance Name |
---|---|
1 | Millar-2Shift-DATA1.1 |
2 | Millar-2Shift-DATA1 |
3 | Ozkarahan |
4 | Musa |
5 | Azaiez |
6 | QMC-1 |
7 | LLR |
8 | SINTEF |
9 | ikegami-3Shift-DATA1.2 |
10 | ikegami-3Shift-DATA1.1 |
11 | ikegami-3Shift-DATA1 |
12 | ikegami-2Shift-DATA1 |
13 | GPOST-B |
14 | BCV-4.13.1 |
15 | GPOST |
16 | Valouxis-1 |
17 | WHPP |
18 | BCDT-Sep |
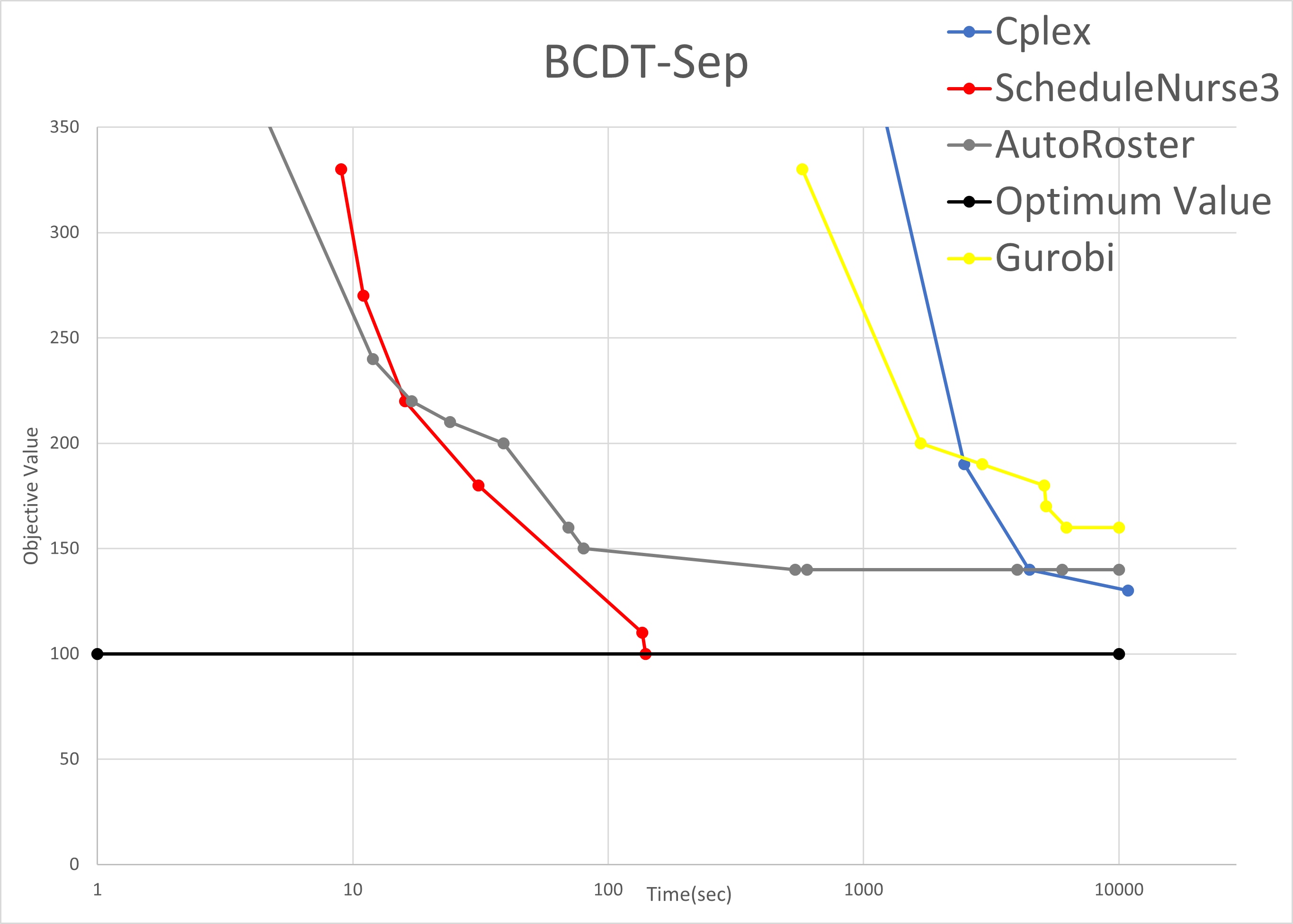
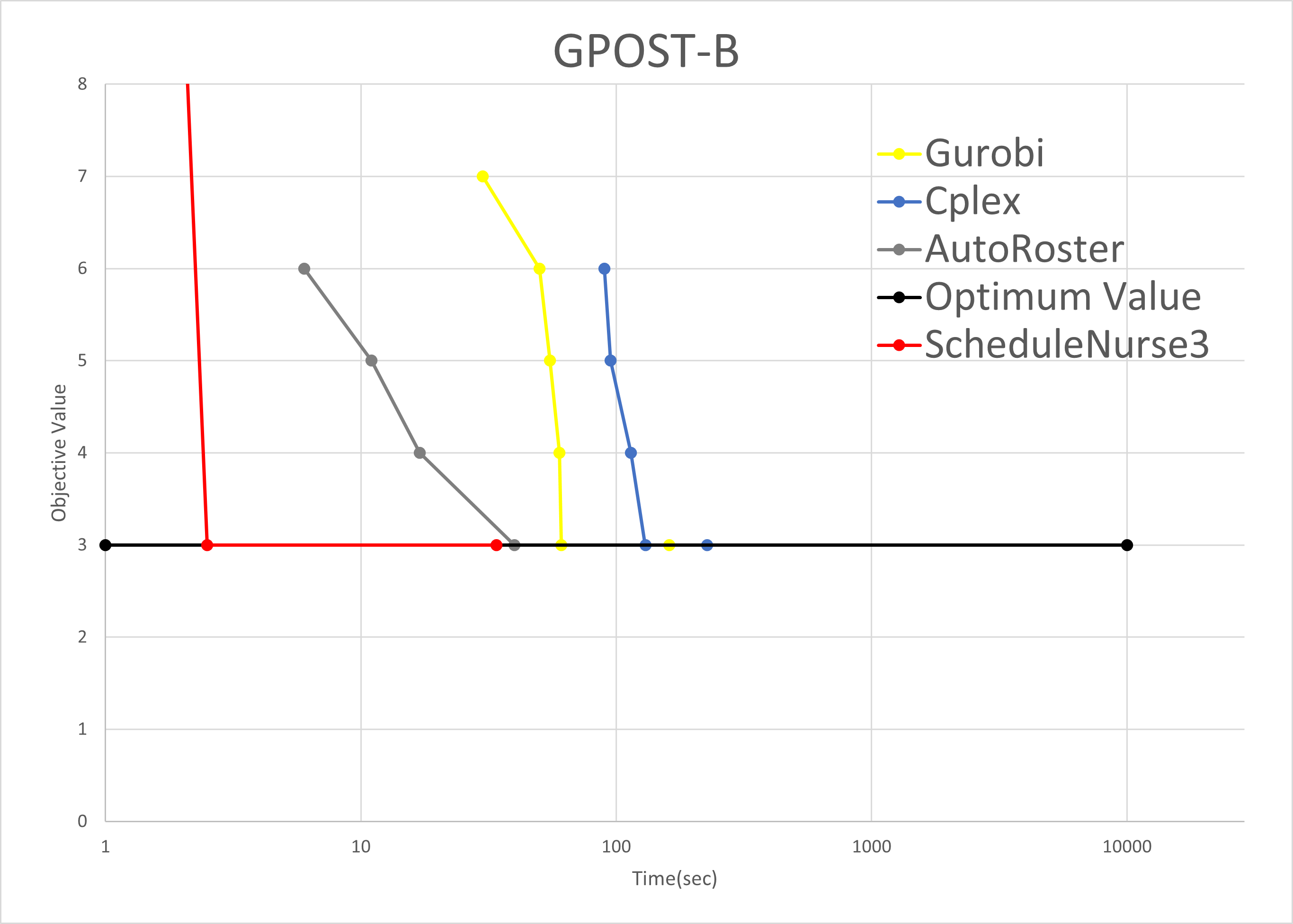
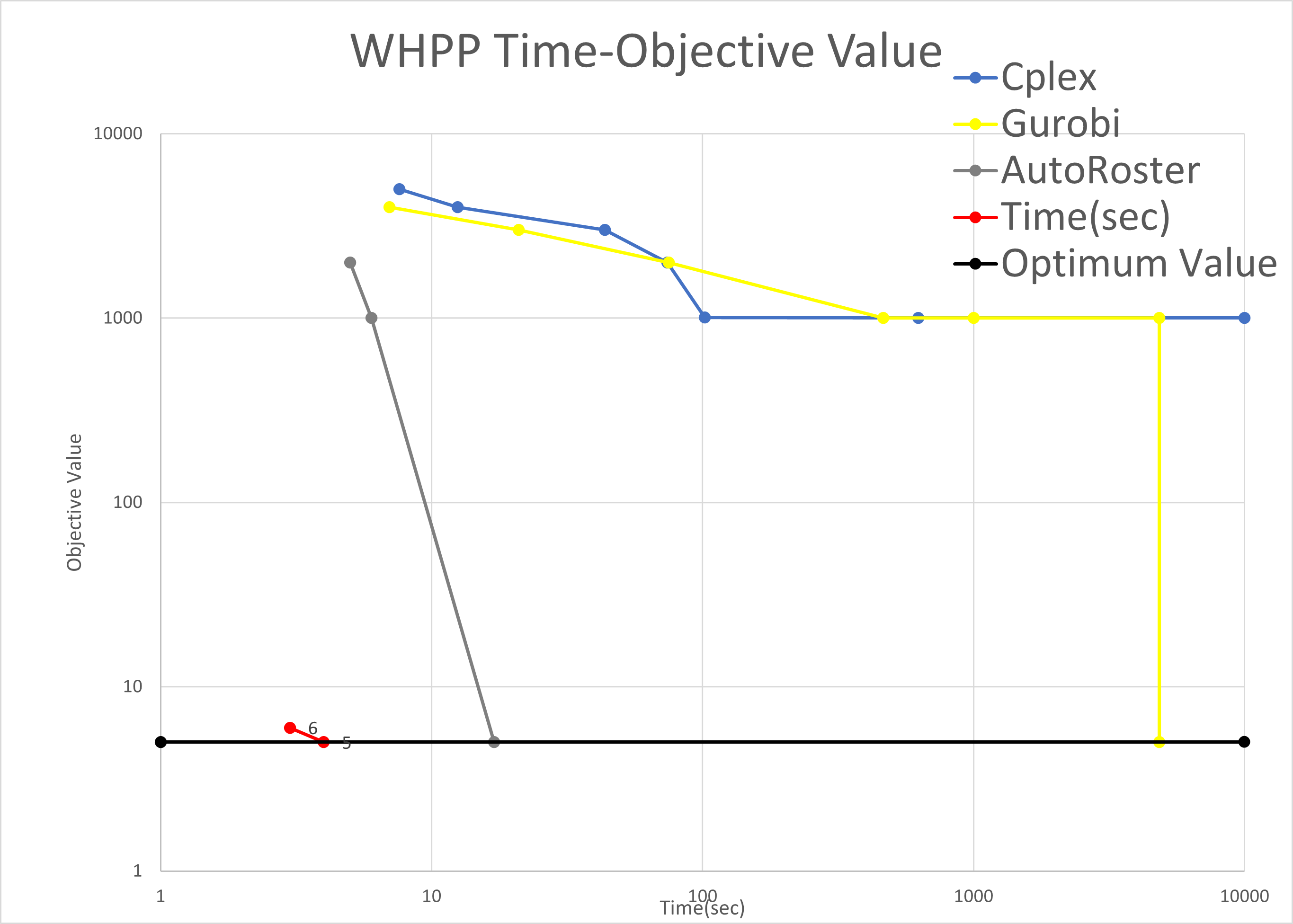
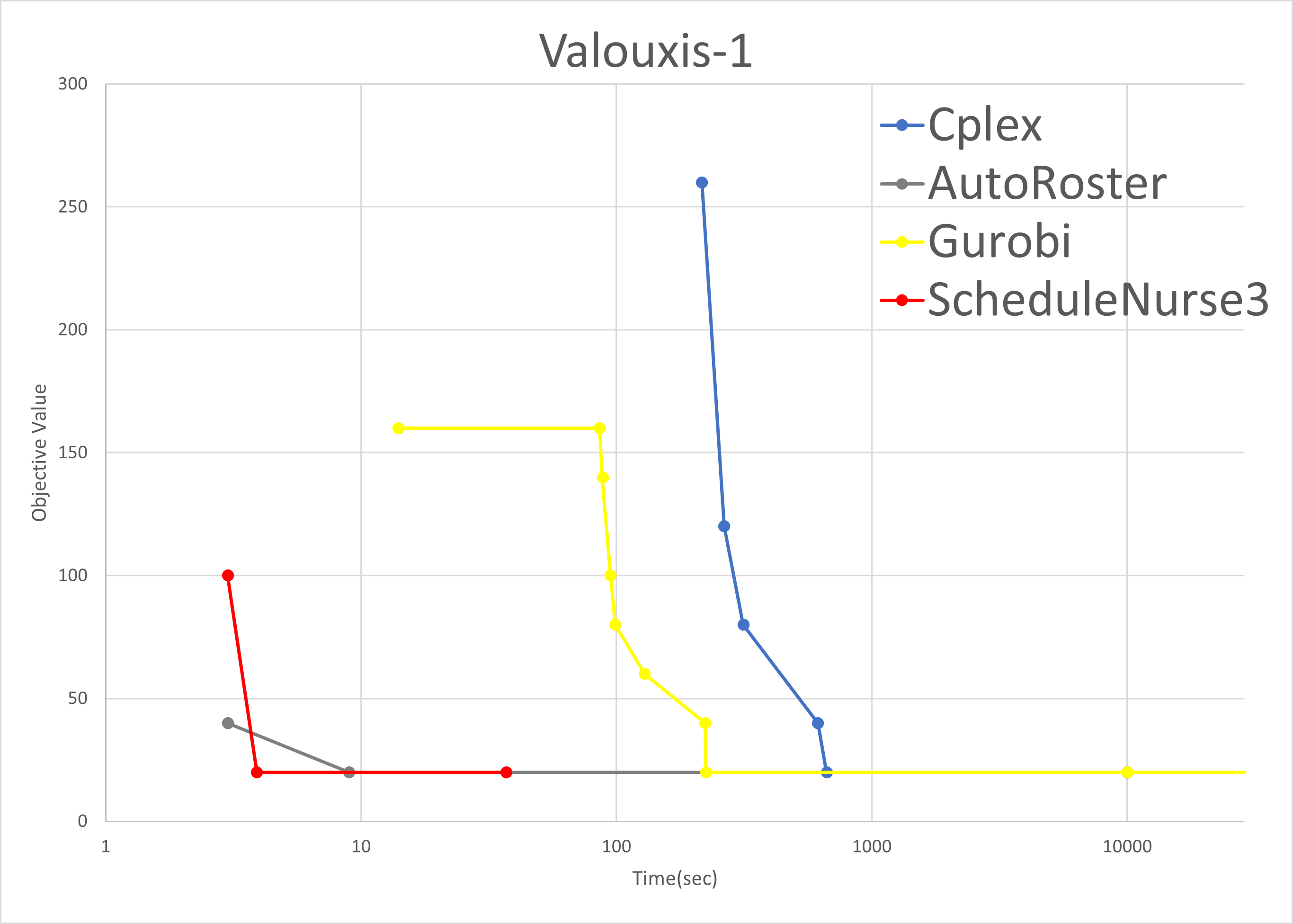
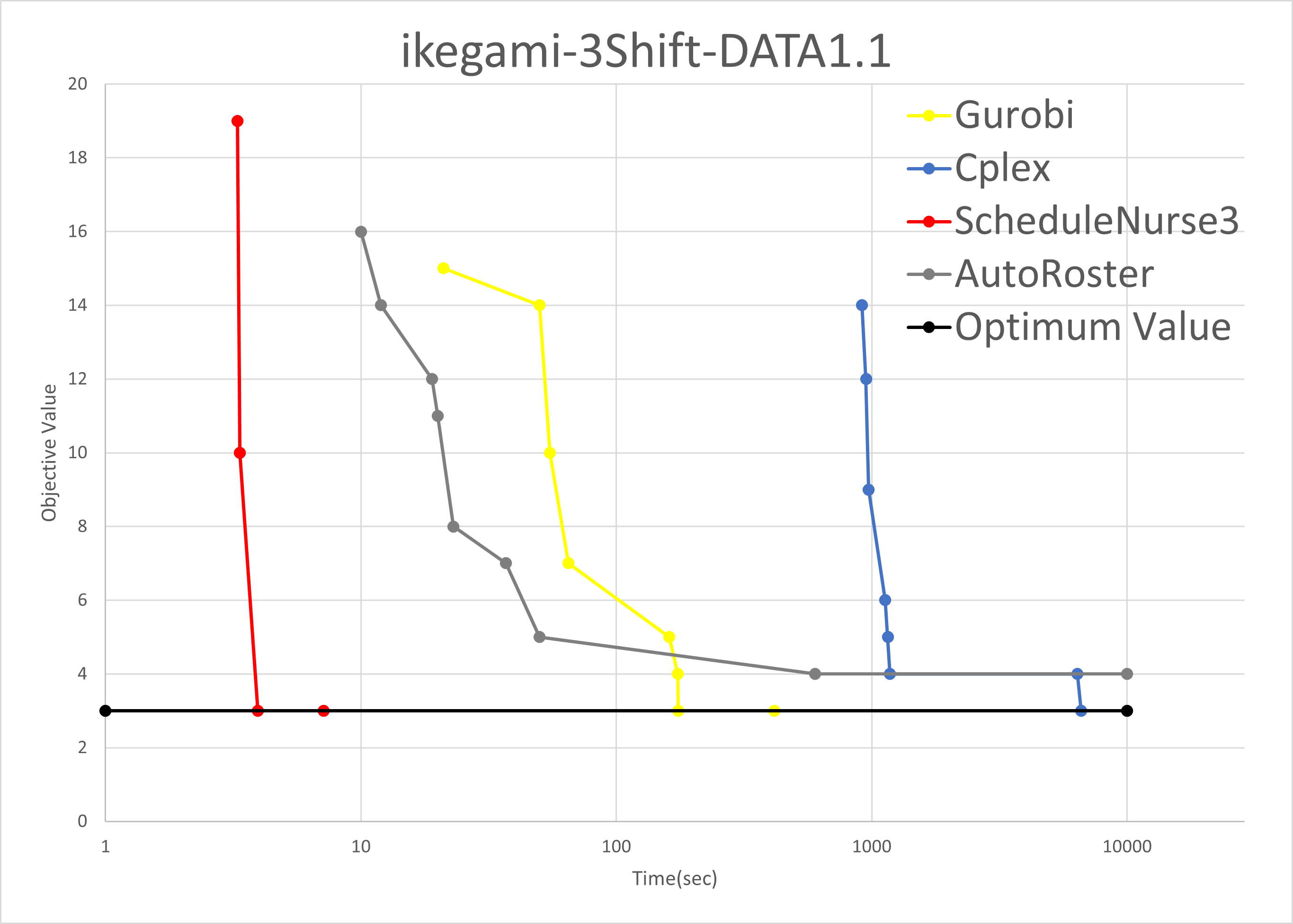
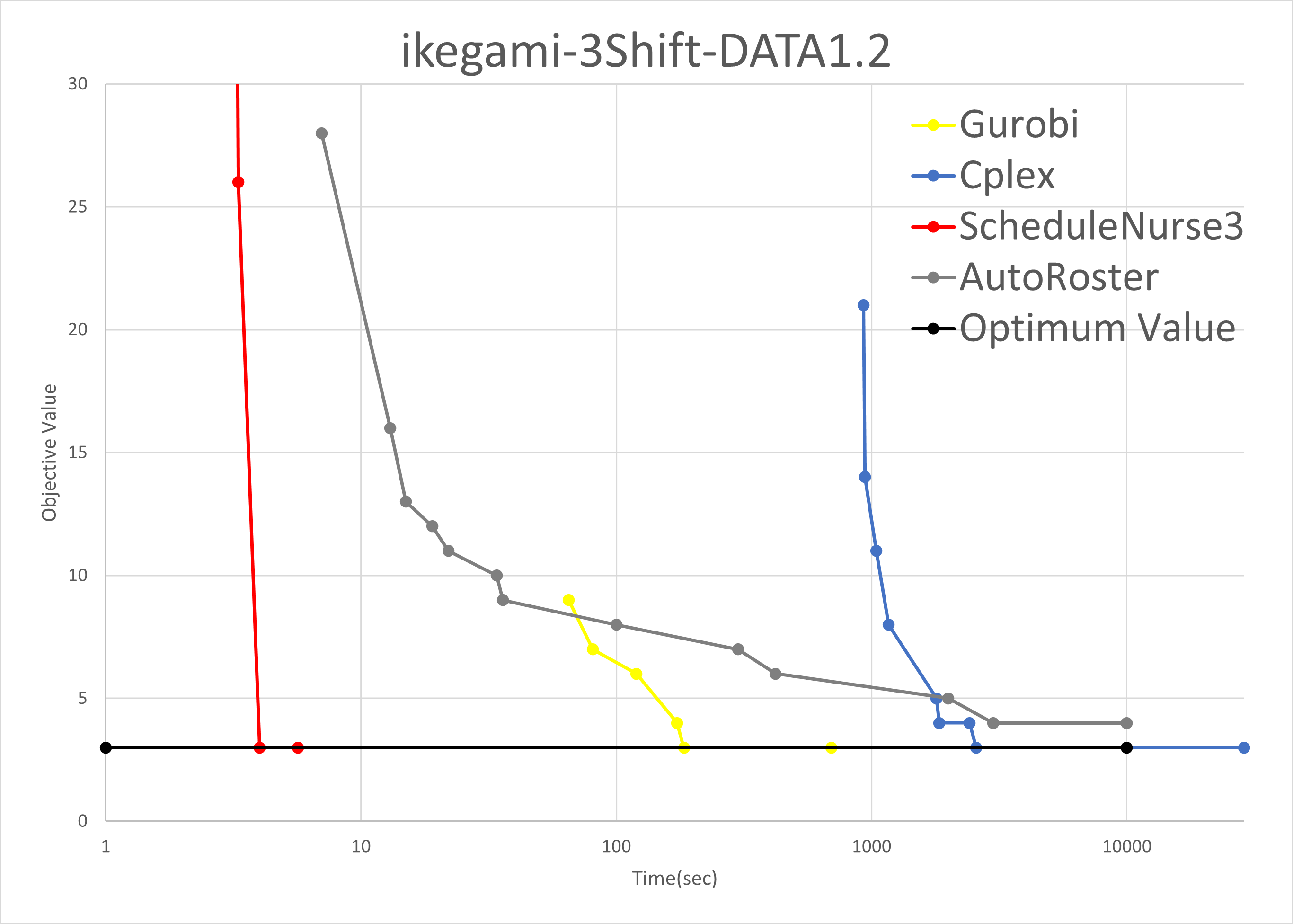
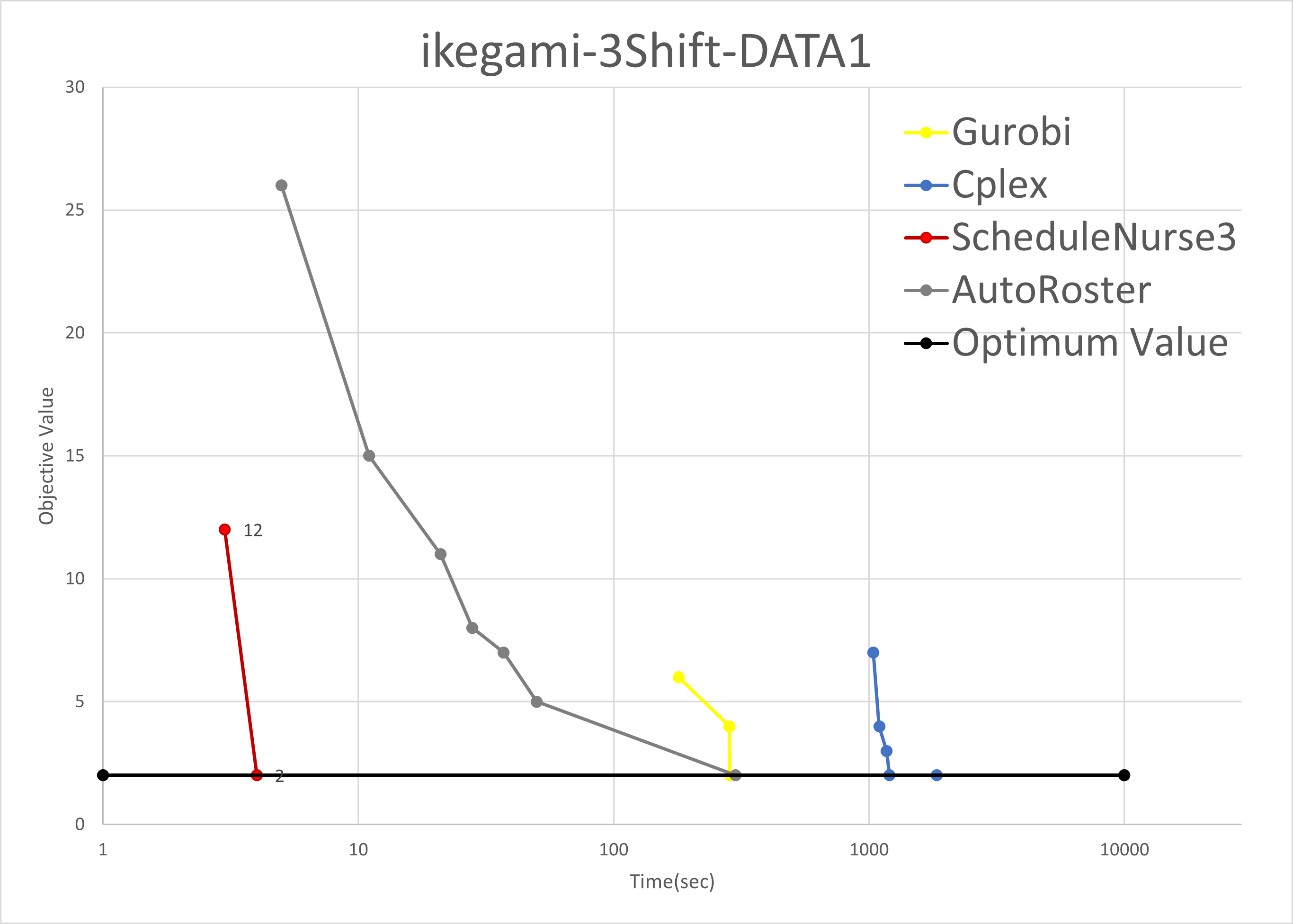
Environment
Solver | Version | Machine |
---|---|---|
Gurobi | Gurobi Optimizer version 9.5.0 build v9.5.0rc5 | NEOS SERVER |
AutoRoster | RosterViewerDemo4.3.5 Branch and Price | Ryzen 5800X 64GB |
Cplex | IBM(R) ILOG(R) CPLEX(R) Interactive Optimizer 20.1.0.0 | NEOS SERVER |
Schedule Nurse3 | Algorithm3 | Ryzen 5800X 64GB |
References
-
Asta, S., Özcan, E., and Curtois, T. A tensor based hyper-heuristic for nurse rostering. Knowledge-based systems, 2016. 98: p. 185-199.
-
Burke E.K. and T. Curtois. New Approaches to Nurse Rostering Benchmark Instances. European Journal of Operational Research, 2014. 237(1): p. 71-81. pdf.
-
Solos, Ioannis P., Ioannis X. Tassopoulos and Grigorios N. Beligiannis. A Generic Two-Phase Stochastic Variable Neighborhood Approach for Effectively Solving the Nurse Rostering Problem. Algorithms, 2013. 6: p. 278-308.
0 件のコメント:
コメントを投稿